In the heart of Stockholm at the dawn of a typical workday Erik Johansson, an experienced asset manager, looked out at the cityscape from his high-rise office. He had always enjoyed the predictability of his routine, the familiarity of his responsibilities – overseeing the company’s assets, from machinery to real estate. But today was different. Erik found himself on the cusp of a revelation that would challenge his understanding and shift his perspective. This was the beginning of his journey.
Erik had recently been introduced to the concept of intangible assets, those elusive often overlooked assets that held extraordinary value in the digital age.
He began to realize the wealth of data his company generated and processed daily, the intellectual property they developed, and the reputation they had built, all contributed to a vast reservoir of intangible assets.
Erik felt like he was standing at the mouth of a labyrinth, equipped with a map that only showed the physical world. The intangible assets were like a hidden dimension, waiting to be discovered and managed. He realized his role as an asset manager was about to expand significantly. All he needed was a new multi-dimensional map, and a language to describe the coordinates and their relations.
His call to adventure had arrived. Exploring Digital Twins and Operational Reference Models.
Embracing this new challenge, Erik began to explore the realm of intangible assets. He immersed himself in learning about digital twins – virtual replicas that could replicate the performance of physical entities in real-time. He learned how his enterprise with the help of these digital twins could begin to predict potential issues, optimize resources and reduce costs. The more he discovered, the more he realized the potential they held for revolutionizing asset management. Soon he encountered a new capability which he hadn’t seen before. An Operational Reference Model (ORM) which was a framework that bridged the gap between physical machines, processes, humans, and entire production lines. ORM came coupled with capabilities to manage and govern taxonomies and ontologies which in turn provided structure and context to data. Erik began to see the possibility of a holistic view of his assets.
But he persisted, his conviction unwavering. He demonstrated the benefits of managing intangible assets in a new way, providing new and unseen capabilities and dependencies. He also experimented with scenarios for the future, what if’s and could be’s, all supported by the ORM to ensure a holistic perspective.
This was his road of trials. Erik faced initial resistance from his team, a natural reaction to change.
With the help of external ORM specialists, Erik integrated ORM with taxonomies and ontologies, into his asset management strategy. Erik’s transformation was underway. Armed with this newfound knowledge and the tools to manage both tangible and intangible assets, Erik began to make significant strides. Together with his architects and IT department he formed a true transformation team. The team enabled the enterprise to begin using the power of digital twins to optimize resources, cut costs, and improve efficiency. He adopted ORM to gain a comprehensive understanding of his company’s assets and orchestrated a common language for his business. This enabled a new era of informed decision-making in the enterprise.
Across the enterprise he saw tangible benefits, as intangible assets were finally recognized and managed effectively. Erik had crossed the threshold.
He had journeyed from a conventional asset manager, overseeing physical assets, to a pioneering figure embracing the complex world of intangible assets. His role had evolved and with it he was driving his company towards a more innovative, integrated, and effective approach to asset management.
In the words of Benjamin Franklin, “An investment in knowledge pays the best interest.” Erik’s journey was a testament to this. His investment in the knowledge of intangible assets and their management has paved the way for continued innovation and success within his organization. His journey has transformed him from an asset manager to a strategic guide (and hero), navigating his organization through the complex landscape of the digital age.
As Erik’s journey reaches its conclusion, he brings back with him a wealth of insights and experiences. He shares his new understanding with his team which helps fostering a culture that recognizes the value of intangible assets. His story inspires others in his field to embark on their own journeys of discovery and transformation.
Erik’s journey is a testament to the evolving role of asset managers in today’s rapidly digitizing world.
It illustrates the importance of integrating modern digital tools and methodologies, such as digital twins and capabilities like an Operational Reference Model, to gain a 360-degree perspective on both tangible and intangible assets.
Through Erik’s transformation we see the emergence of a new era in asset management – an era where intangible assets are recognized for their immense value and potential.
An era where asset managers evolve from custodians of physical assets to strategic guides, navigating the labyrinth of intangible assets.
Erik’s story is just the beginning. There are countless other asset managers out there, standing on the cusp of their own journey.
In this evolving landscape, the dialogue continues: Are you ready to embark on this journey? Are you prepared to expand your understanding and evolve your asset management strategies? Are you ready to embrace the paradox of intangible assets and harness their potential? The future of asset management is here. Let us embrace it, evolve with it, and drive our organizations towards continued innovation and success.
The importance of intangible assets
As Erik’s journey illuminates the importance of intangible assets, such as data and information about our capabilities, has increased exponentially. Today data and information has become a key driver of growth. Companies like Facebook and Google rely heavily on user data to generate revenue. Data is a key factor in driving business decisions, innovation, and customer experiences. Companies in manufacturing and healthcare has started to understand the importance of information as a way to capture and automate complex knowledge that resides in the minds of people or is embedded in a product, service or process. Like other types of assets, intangible assets are essential to the organization’s success.
Navigating the challenges and complexities of managing intangible assets is crucial to unlocking their full potential. Ensuring data accuracy, data security, and compliance is fundamental to maintain trust and protect company reputation. Ensuring an evolvable operational information model means a common language and true transparency cross the organization to provide the real foundation for a data driven enterprise. This is where the role of an asset manager becomes even more critical.
It involves not just overseeing physical assets but also ensuring that data and information are accessible, accurate and secure
– and has a common point of reference.
Deriving value from intangible assets, like knowledge, information and data, requires managing them with a set of tools designed and built for purpose. It’s not only big data, but also small data. Metadata is small data which provides data with context, it also provides context in terms of relations to other data. Without it, asset management is hard and only available to a small group of people within a company. Rapid advancements in technology, including the rise of artificial intelligence and machine learning, have shifted the value landscape. These advancements have opened up new opportunities for businesses to leverage data.
Intangible assets, particularly data, need specific models, methods, and technologies to forecast performance and simulate decision-making processes. This is where digital twins and ORM come into play. Digital twins are virtual representations of assets (both physical and virtual) used for monitoring, simulation, and optimization. The ORM on the other hand provides the structure necessary to succeed with data management.
To illustrate, consider a wind turbine farm using digital twins for each turbine. The digital twins collect data on the turbines’ performance, such as wind speed, energy output, and temperature. By analyzing this data in real-time, the digital twin can identify potential issues or inefficiencies, such as a misaligned blade or an overheating gearbox, before they lead to a critical failure. ORM, in the meantime, provides a framework for managing these data points, ensuring that they are accurately captured, categorized, and accessible when needed. This allows to learn from other wind turbine farms and copy best practice with a different configuration of a wind farm (location, layout and manufacturer).
The use of digital twins and ORM in asset management is just one part of the equation. It’s also important to recognize that intangible assets like Spotify’s vast data on listening habits, Tesla’s patents and technology, and Netflix’s customer data all contribute to their respective company’s competitive edge. As such, asset managers today need to factor in these intangible assets when developing and implementing asset management strategies.
Erik’s journey underscores the importance of leveraging modern digital tools and methodologies to manage both tangible and intangible assets effectively. His transformation from a traditional asset manager to a strategic guide navigating the complex landscape of the digital age is indicative of the shift happening within the asset management industry.
As we continue to evolve in this new era of asset management, it’s essential to engage in dialogue and share insights. Are you ready to expand our understanding and evolve our asset management strategies? Are you prepared to embrace the paradox of intangible assets and harness their potential? Let’s continue the conversation and drive our organizations towards innovation and success in the digital age.
More on this topic: ISO TC 251 Asset Management Plenary Session
Other suggested blogs: Democratization of Data, A Game Changer for Productivity and Business Intelligence
/Daniel Lundin, Head of Product & Services
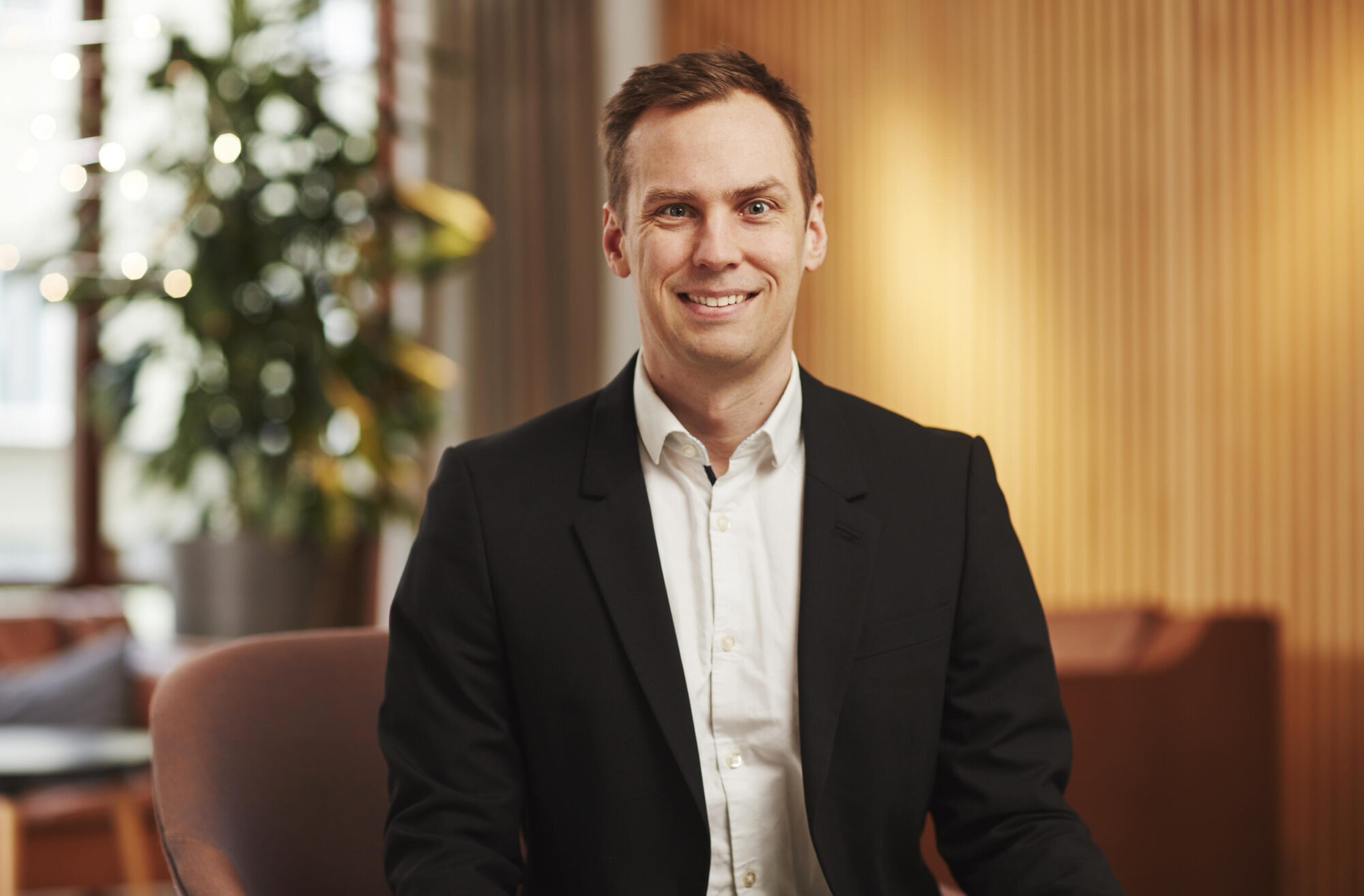